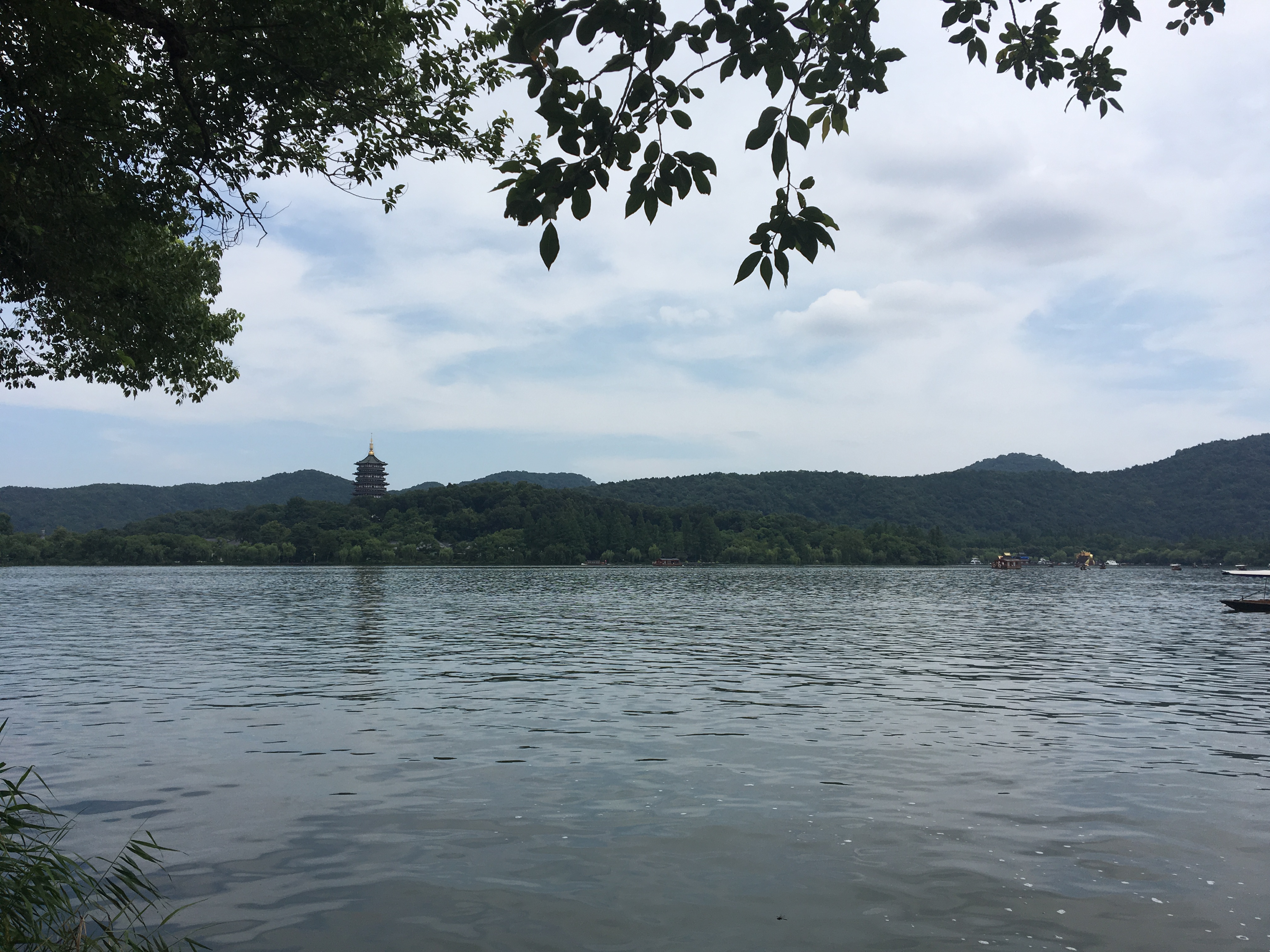
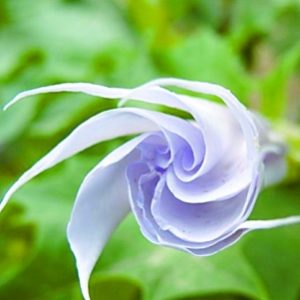
Datura
MemberForum Replies Created
-
From SAS Support Website:
Problem Trying to Reference a SYMPUT-Assigned Value Before It Is Available.
One of the most common problems in using SYMPUT is trying to reference a macro variable value assigned by SYMPUT before that variable is created.
The failure generally occurs because the statement referencing the macro variable compiles before execution of the CALL SYMPUT statement that assigns the variable’s value. The most important fact to remember in using SYMPUT is that it assigns the value of the macro variable during program execution, but macro variable references resolve during the compilation of a step, a global statement used outside a step, or an SCL program. As a result:
* You cannot use a macro variable reference to retrieve the value of a macro variable in the same program (or step) in which SYMPUT creates that macro variable and assigns it a value.
* You must specify a step boundary statement to force the DATA step to execute before referencing a value in a global statement following the program (for example, a TITLE statement). The boundary could be a RUN statement or another DATA or PROC statement. For example:
data x;
X='December';
call symput('var', X);
Run;
proc print;
title "Report for &var";
run; -
Example: How to find all the Friday dates in a given month ??
A could be any given date, Friday_Date is the dates of all the Fridays in the same MONTH as A.
data BBB;
A="06JAN2019"d;
B=intnx("week", A, 0);
C=intnx("day", B, 5);
Do I=-5 to 5;
Friday_Date=C+I*7;
format B C Friday_date date9. ;
if month(Friday_Date)=month(A) then output;
end;
run;Summarize the total number of Friday dates in a month
data C;
set BBB END=EOF NOBS=N;
DD=Friday_date-A;
If DD GE 0;
run;
Data D;
set C End=EOF NOBS=N;
call symput("Num_Friday", put(N,1.));
%put Number of Friday in this month is *&Num_friday*;
run;
Proc sql;
select Friday_date INTO: Friday1-:Friday&Num_friday
from D;
quit;
%put _user_;-
This reply was modified 3 years, 12 months ago by
Datura.
-
This reply was modified 3 years, 12 months ago by
-
Look Ahead — Opposite of LAG function
We can use SET statements to construct the opposite of the LAG function, namely a “look ahead”.Data A;
Input name $ sales;
cards;
Alice 100
Jenny 265
Lynne 785
Zane 963
Mary 612
;
run;Data CCC;
Set A (firstobs=2 rename=(sales=Next_sales) ) END=EOF NOBS=I;
Set A;
DIF=Next_sales - sales;
Output;
If EOF then do;
Set A point=I;
Next_sales=.;
DIF=Next_sales - sales;
Output;
End;
Run;Watch out at the end: the first SET A statement will signal EOF=1 first, so that the last observation in the 2nd SET A will not be read in, unless we use the IF EOF statement to execute the 2nd SET A twice. The explicit output statements are necessary.
-
Call SYMPUT rountine
Call SYMPUT is a call routine in SAS, it generate a macro variable in a DATA step and puts the macro variable in the most local non-empty symbol table. A symbol table is nonempty if it contains the following:
1. a value;
2. a computed %GOTO (A computed %GOTO contains % or & and resolves to a
label.)
3. the macro variable &SYSPBUFF, created at macro invocation time.CALL SYMPUTX Routine: It is an upgraded version of Call Symput with below enhanced features.
1. Assigns a value to a macro variable, and removes both leading and trailing
blanks.2. It has the Symbol Table argument which can be used to specify a macro variable to be local or global.
Call SymputX ("City", "Chicago", "G/L/F");
Symbol-table: G, L, F.
It specifies a character constant, variable, or expression. The value of symbol-table is not case sensitive. The first non-blank character in symbol-table specifies the symbol table in which to store the macro variable. The following values are valid as the first non-blank character in symbol-table:
G: specifies that the macro variable is stored in the global symbol table, even if the local symbol table exists.
L: specifies that the macro variable is stored in the most local symbol table that exists, which will be the global symbol table, if used outside a macro.
F: specifies that if the macro variable exists in any symbol table, CALL SYMPUTX uses the version in the most local symbol table in which it exists. If the macro variable does not exist, CALL SYMPUTX stores the variable in the most local symbol table.Note: If you omit symbol-table, or if symbol-table is blank, CALL SYMPUTX stores the macro variable in the same symbol table as does the CALL SYMPUT routine.
Attributed to these enhanced features, therefore I strongly recommend to use Call SymputX rather than Call Symput in work.
-
This reply was modified 3 years, 12 months ago by
Datura.
-
This reply was modified 3 years, 12 months ago by
-
### 3) Test on means of Sales or other numeric variables by Student t-test and ANOVA.
import scipy.stats as stats
### Two sample t-test
summary =impute.pivot_table('sales',
columns=['credit'],
aggfunc='mean',
margins=False)tStat, p_value = stats.ttest_ind(impute[impute['credit']==0 ]['sales'],
impute[impute['credit']==1 ]['sales'],
equal_var = False,
nan_policy='omit' )
#run independent sample T-Test
print("t-Stat:{0}, p-value:{1}".format(tStat, p_value))
# We cannot reject H0 therefore conclude the sales are same for good and bad credit people.### Multiple sample ANOVA test. We look into the deactivated people only. ###################
summary =impute.pivot_table('sales',
columns=['reason'],
aggfunc='mean',
margins=False)### we need to drop null values on analysis variable, otherwise we will get nan results.
impute.isnull().sum()
sub=impute[ (sub['reason'] !=' ') & (pd.notnull(impute['sales'] ) ) ]FStat, p_value = stats.f_oneway( sub[ sub['reason']=='COMP']['sales'],
sub[ sub['reason']=='DEBT']['sales'],
sub[ sub['reason']=='MOVE']['sales'],
sub[ sub['reason']=='NEED']['sales'],
sub[ sub['reason']=='TECH']['sales'] )print("F-Stat: {0}, DOF={1}, p-value: {2}".format(FStat, len(sub)-1, p_value))
# Since p-value<0.05, we reject H0 and conclude that the sales means
# significantly differ among people with different deactivation reasons.### Note: From ANOVA analysis above, we know that treatment differences are statistically significant,
### but ANOVA does not tell which treatments are significantly different from each other.
### To know the pairs of significant different treatments, we will perform multiple
### pairwise comparison (Post-hoc comparison) analysis using Tukey HSD test.#################### Tukey’s multi-comparison method #########################
# This method tests at p <0.05 (correcting for the fact that multiple comparisons
# are being made which would normally increase the probability of a significant
# difference being identified). A results of ’reject = True’ means that a significant
# difference has been observed.
# load packages
from statsmodels.stats.multicomp import (pairwise_tukeyhsd, MultiComparison)### class statsmodels.sandbox.stats.multicomp.MultiComparison(data, groups, group_order=None)
sub.info()MultiComp = MultiComparison(sub['sales'], ### analysis variable
sub['reason'].replace(' ', ' NA'), ### group variable
group_order=None) ### group_order: the desired order for the group mean results to be reported in.
results=MultiComp.tukeyhsd().summary()
results
############################ End of Program. #################################-
This reply was modified 4 years ago by
Datura.
-
This reply was modified 4 years ago by
-
-
Easy. Create a ID say numeric X by a do loop first, 1,2,3….. then convert it to char and pad with leading zeros using Z6. format. Finally, combine them together. You get it?
Data AAA;
Do X=1 to N;
ID= “aa59” II put(X, Z6.);
Output;
End;
Run; -
However, if we want to call the custom function manually like below, and we also want to add a new column for the column name, we have to use the as_label function to achieve it.
cover<- function(df, x) {mv_x<- enquo(x)
df %>%
proc_freq(0, !!mv_x) %>%
mutate(var=as_label(mv_x)) %>%
select(value, freq, cum_freq, pct) }as_label() transforms R objects into a short, human-readable description. You can use labels to:
1) Display an object in a concise way, for example to labellise axes in a graphical plot.
2) Give default names to columns in a data frame. In this case, labelling is the first step before name repair.
Unlike as_label(), as_string() is a well defined operation that guarantees the roundtrip symbol -> string -> symbol. In general, if you don’t know for sure what kind of object you’re dealing with (a call, a symbol, an unquoted constant), use as_label() and make no assumption about the resulting string.
If you know you have a symbol and need the name of the object it refers to, use as_string(). For instance, use as_label() with objects captured with enquo() and as_string() with symbols captured with ensym().
-
This reply was modified 4 years, 2 months ago by
Datura.
-
This reply was modified 4 years, 2 months ago by
-
再说一下 data scientists 面试的要点:
(1) 有”一定” 的技术能力,Python + big data (比如Hive/SQL)。R 也可以,但是很多面试题用 R 来做恐怕有些困难,会Python是很有帮助的。不需要啃算法书,CS面试那种算法题不会考,我们只需要知道你有独立 handle data 的能力,遇上事情能有技术能力 unblock你自己,光会SQL是不行的。
(2) 有 “极好” 的分析能力。 这是analysts的本质,也是面试的重点。
给你一个问题(open ended),看能不能 think analytically and structurally。随便举个例子:
怎么用FB数据分析日本核电站爆炸的影响?这里要自己 formalize这个问题,提出假设,思考可以用哪些数据,怎么分析,没有某些数据怎么替代等等一系列步骤。
(3) 有”极好”的product sense。因为实际工作中,很多时候没人问你问题,你要自己问问题,问正确的问题,需要对产品有很好的理解。这里也举个例子:
假如印度的某个城市停电三天,你觉得对FB会有哪些影响?这些影响对Twitter会如何?回答这个问题,先得对行业和产品有一定了解,否则根本无从下手。
当然一些其他的东西,比如对数字的敏感度,交流的能力等等很重要,但是那些都表现在上面这些回答当中。
很大程度上,FB 的 data scientists 需要的是通才, 而不是专才,从上面这些问题就能看的出来。这也是为什么我们招进来的人什么背景的都有,背景可以千差万别,但是上面三条都一定满足。对于学统计的人,尤其是对互联网行业比较陌生的,可能在 product sense 方面会有些challenge。我们也面试过很多统计PhD,有些人回答很好,有些人就一塌糊涂,最后的差别并不在于统计,而是分析头脑和 product sense。
这里要说一点,大多数中国人都比较适合回答 close-ended questions (这个和咱们的教育背景有关), 纠结于”怎么分析”?很多时候遇上 “分析什么” 这种 open-ended questions 反而不知如何下手。
最后说点题外话。我来FB之前在Google工作了7年半,Google是个给钱多还不累的地方;FB可完全不是,FB比 Google忙的多的多的多的多,整个公司都是这个文化。 对于 mediocre performer,Google 更 tolerant,而 FB 很多就直接fire了。所以追求 work life balance 的, 基本就不要考虑FB了。
Google是很好的公司,也有很多牛人,但也有很多不干活的人在公司的各个角落。FB可以说基本没有,至少整个 analytics org 一百多人,我还没发现任何一个人有任何一点” 混”的迹象。Analytics 从 director到 manager 到 IC,都是要做 IC活 的,光管人耍嘴皮子,在FB是吃不开的。
-
This reply was modified 4 years, 2 months ago by
Datura.
-
This reply was modified 4 years, 2 months ago by